- Home
- Blog
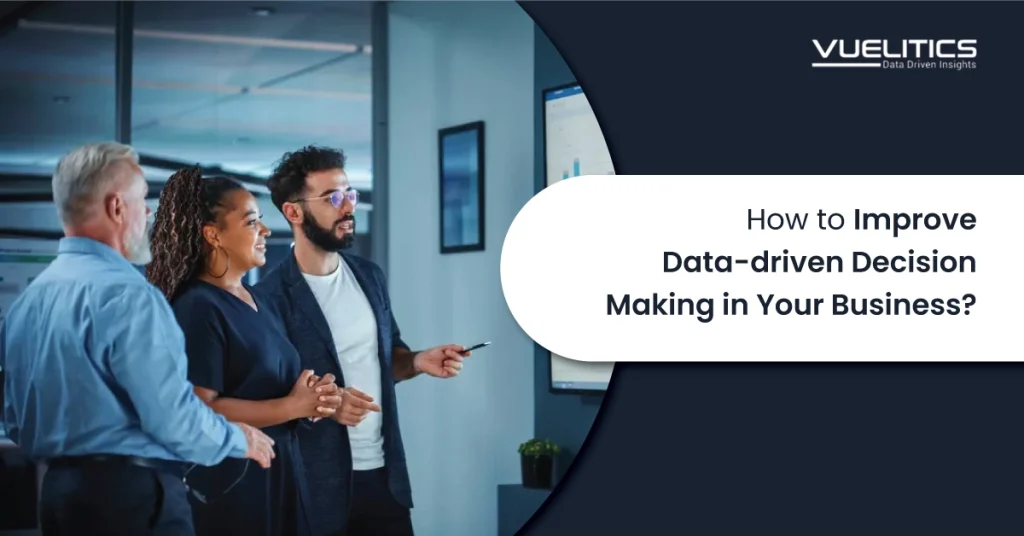
How to Improve Data-driven Decision Making in Your Business?
Improve data-driven decision-making in your business.
In today’s business landscape, data is the driving force behind strategic decision-making. Companies that leverage data to inform their decisions are better positioned to achieve growth and innovation. The Data-Driven Decision Making (DDDM) process allows businesses to use data to make decisions that are faster, more accurate, and more cost-effective. This process involves transforming raw data into actionable insights that align with a company’s goals and objectives.
What is data-driven decision making?
Data driven decision making (DDDM) is the process of making decisions based on the interpretation of data and analysis instead of observation, intuition, or personal experience. This helps identify patterns and trends to gain insights or business strategies. Using several key components of data to make decision making, companies can develop frameworks and continuously monitor the output to implement necessary changes. Here is why companies need DDDM;
- Improved Efficiency: Streamlines business processes, reducing waste and operational costs.
- Enhanced Accuracy: Reduces errors by relying on factual data rather than subjective judgments.
- Competitive Advantage: Provides strategic insights that give businesses an edge over competitors.
- Better Forecasting: Allows for more accurate predictions of future trends and market conditions.
- Increased Transparency: Promotes accountability and openness within the organization.
The Data-Driven Decision-Making Process
Mastering the art of data-driven decision making involves a systematic and iterative approach. Here are the key steps in the DDDM process:
1. Understanding the Company’s Vision and Goals:
- Aligning Objectives: Begin by aligning data initiatives with the company’s broader goals and strategic objectives. This ensures that data efforts are focused on achieving meaningful business outcomes.
- Defining Success Metrics: Establish clear metrics and key performance indicators (KPIs) that will measure the success of data-driven initiatives. These metrics should be specific, measurable, achievable, relevant, and time-bound (SMART).
2. Identifying and Collecting Data Sources:
- Internal Data Sources: Collect data from internal systems such as CRM, ERP, and HR systems. This data includes sales figures, customer interactions, employee performance, and financial records.
- External Data Sources: Incorporate data from external sources such as market research reports, social media, industry benchmarks, and third-party databases. This provides a broader context for analysis.
- Data Integration: Combine data from multiple sources to create a comprehensive dataset. This step often involves data warehousing and ETL (Extract, Transform, Load) processes to ensure data consistency and accuracy.
3. Organizing and Preparing the Data:
- Data Cleaning: Clean the data to remove duplicates, correct errors, and handle missing values. Data quality is critical to ensure accurate analysis and reliable insights.
- Data Structuring: Organize the data into a structured format that is easy to analyze. This may involve categorizing data, creating relational databases, or using data lakes for unstructured data.
- Data Enrichment: Enhance the dataset by adding additional context or derived variables. For example, calculate customer lifetime value (CLV) or segment customers based on behavior patterns.
4. Analyzing the Data:
- Descriptive Analytics: Use descriptive analytics to summarize and understand historical data. Techniques such as data visualization, dashboards, and reporting tools help identify trends and patterns.
- Diagnostic Analytics: Perform diagnostic analytics to understand the causes of past performance. This involves statistical analysis, correlation studies, and root cause analysis.
- Predictive Analytics: Employ predictive analytics to forecast future outcomes based on historical data. Techniques include regression analysis, time series forecasting, and machine learning models.
- Prescriptive Analytics: Utilize prescriptive analytics to recommend actions based on the analysis. Optimization algorithms, simulation models, and decision trees are common tools used in this stage.
5. Drawing Conclusions and Making Decisions:
- Insight Generation: Translate the analysis into actionable insights that align with business objectives. This involves identifying key findings, implications, and opportunities for improvement.
- Decision Making: Use the insights to make informed decisions. This could involve strategic planning, operational adjustments, or tactical actions to achieve desired outcomes.
- Implementation: Execute the decisions and implement the recommended actions. Ensure that there is a clear plan for execution, including timelines, responsibilities, and resources.
6. Monitoring and Evaluating Results:
- Continuous Monitoring: Continuously monitor the outcomes of the decisions to assess their impact. Use real-time dashboards and key metrics to track performance.
- Feedback Loop: Establish a feedback loop to refine and improve the decision-making process. Collect feedback from stakeholders, analyze the effectiveness of actions, and make necessary adjustments.
- Iterative Improvement: Treat DDDM as an iterative process. Regularly review and update data sources, analytical models, and decision frameworks to adapt to changing business environments and new insights.
Benefits of Data-Driven Decision-Making
Incorporating data-driven decision-making (DDDM) into your business strategy can significantly enhance your operations and outcomes. Here are some of the key benefits:
Increased Confidence in Decision-Making
Collecting and analyzing data makes it easier to make confident decisions about various business challenges, whether it’s launching a new product, modifying your marketing strategy, or entering a new market.
- Benchmarking Current Performance: Data allows you to establish benchmarks for your current operations, helping you understand the potential impact of your decisions.
- Objectivity and Logic: Unlike gut instincts or intuition, data is logical and concrete. This objectivity helps remove subjectivity from the decision-making process, instilling greater confidence in your choices.
- Commitment to Strategy: With data backing your decisions, your organization can fully commit to a vision or strategy without excessive concern about making the wrong choice.
While data-driven decisions are generally more accurate, they are not infallible. Any decision based on flawed data collection or interpretation can lead to incorrect conclusions. Therefore, it’s essential to regularly measure and monitor the impact of your decisions.
Enhanced Proactivity
Initially, DDDM may be reactionary—analyzing data to respond to current trends or issues. However, with enough practice and the right data, it can become a proactive tool.
- Identifying Opportunities: Proactively leveraging data can help you identify business opportunities before your competitors do.
- Detecting Threats Early: It can also enable you to detect potential threats early, allowing you to address them before they become significant issues.
Cost Savings
One of the most impactful benefits of becoming data-driven is the potential for cost savings. According to a survey of Fortune 1,000 executives conducted by NewVantage Partners for the Harvard Business Review, many organizations have realized substantial value from initiatives aimed at reducing expenses through data analysis.
- Expense Reduction: Of the organizations that began projects designed to decrease expenses, more than 49 percent reported seeing value from their initiatives.
- Mixed Results in Other Areas: While cost reduction has shown significant success, other initiatives have had more mixed results. Nonetheless, the potential for financial benefits from a data-driven approach is considerable.
Strategies to Improve Data-Driven Decision-Making in Your Business
Businesses need to continuously improve data-driven decision-making by following different strategies. This involves effective collection, analysis, and usage of data.
- Creating a data-driven culture: The first step to follow is to ensure that data-driven culture is being implemented in all areas of the business. This will allow the collective involvement of all the parties.
- Investing in tools and technology: A business must invest in a dynamic and robust data management system to collect, store, and organize the data. You must also use advanced tools to analyze the data and gather insights.
- Leveraging advanced analytics: Businesses can use predictive analytics, Machine Learning, or Natural Language Processing tools to forecast future trends and identify patterns, and outcomes.
- Setting clear objectives: To achieve the data initiatives, you need to define the business goals and objectives clearly. You can also establish KPIs to understand the success of data-driven decision-making initiatives.
Data-Driven Decision-Making Examples
Here are some examples of data driven decision making across industries
- Manufacturing: Predictive maintenance is a data driven approach used in most Manufacturing industries. This approach helps them foresee machinery and equipment failures beforehand. Companies analyze historical data and real-time sensor information to predict when there is a need for maintenance, reduced downtime, and other key factors.
- E-commerce: In the e-commerce sector, personalized recommendations have played a deciding factor in the data-driven decision-making process. It utilizes the behavior analysis of the customers to offer customized suggestions for various products which helps in enhancing the user experience.
- Finance: Businesses utilize various advanced analytics and machine learning options to effectively leverage data-driven strategies. With cybercrime being the top threat to the financial industry, various finance companies leverage big data and AI to analyze customer transaction records in real-time to ensure there are no fraudulent activities recorded.
- Healthcare: Healthcare providers make the best use of patient information, medical history, allergies, and outcomes to create holistic patient care and allocate resources by making informed choices.
Major Challenges & trends in Data-Driven Decision Making
Although businesses benefit hugely from data-driven decision making, there are several challenges that businesses face while implementing this approach. There is a need to understand the challenges and find a clear way to address them successfully.
- Data Accuracy and Quality: The pillar of data-driven decision-making is the quality of the data and its accuracy. Any compromise on the quality of the data will lead to ineffective analysis, ultimately leading to unhealthy decisions. This brings focus to data reliability, which will guide businesses in making informed decisions.
- Data Security and Privacy: Companies must ensure that they comply with regulations like GDPR, CCPA, and HIPAA. Data security measures should also be implemented, like encryption, system updates, and access control.
- Open to change: While implementing data-driven decision-making, resistance to change often becomes inevitable. To come out of this, there should be clear communication of perks and openly addressing key concerns in the organization.
Overcoming Challenges in Data-Driven Decision Making
To address these challenges effectively, businesses can adopt the following practices:
- Data Governance: Implement strong data governance policies to ensure data accuracy, consistency, and security. This includes defining data ownership, establishing data quality standards, and implementing data stewardship roles.
- Training and Development: Invest in training programs to enhance data literacy among employees. This will help them understand the importance of data and how to use it effectively in their roles.
- Collaboration and Communication: Foster a collaborative environment where data insights are shared across departments. Use data dashboards and visualization tools to make data accessible and understandable for all employees.
Trends in Data-Driven Decision Making
Companies need to improve and enhance their resources to make effective decisions. Technology has paved the way for various new trends that provide huge opportunities for growth. Here are the big trends in data-driven decision-making.
- Artificial Intelligence and Machine Learning: AI and ML are extensively used to gain actionable insights. It helps companies fully automate the data analysis process and come up with practical decisions.
- Cloud Computing: Cloud-based storage allows scalability and flexibility for companies gearing up for data-driven decisions.
- Big Data: Implementing big data for data analysis will help companies process large volumes of data under a single roof.
- Natural Language Processing (NLP): There is an increase in the usage of NLP to analyze and convert unstructured data from voice and text. NLP can also be used to understand customer feedback and make decisions based on that opinion.
These challenges and trends often portray the evolving picture of data-based decision-making. There is a continuous need to adapt and invest in technology and processes to make accurate decisions.
Conclusion
When companies leverage data-driven decision-making effectively, they can enhance their operational efficiency, improve the customer experience, and have a competitive advantage. By investing in the current tools, the right talent, and being open to change, companies can effectively transform data and drive growth. To conclude, data-driven decision-making is a necessity for new-age businesses. When companies can embrace it openly, they are one step closer to achieving more agile and efficient organizational growth.
For more information on boosting your business with expert data insights, visit our business intelligence and data analytics services.
Recent Posts
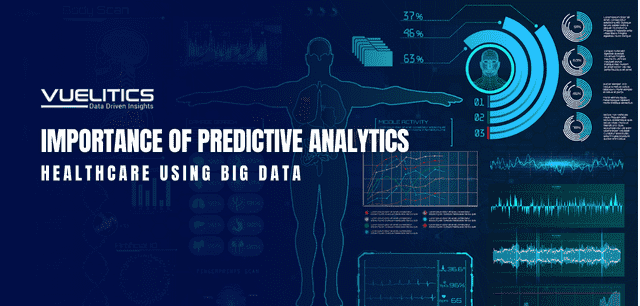
Importance of Predictive Analytics in Healthcare Using Big Data
2 September, 2024
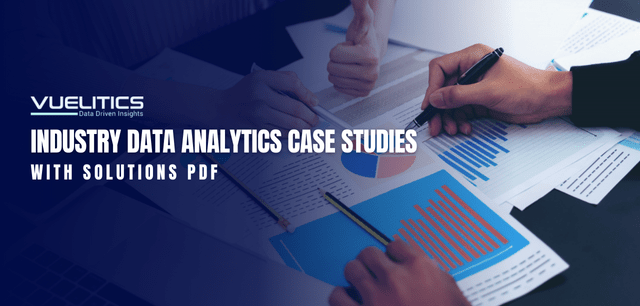
Industry Data Analytics Case Studies with Solutions PDF
29 August, 2024
Post Comments