- Home
- Blog
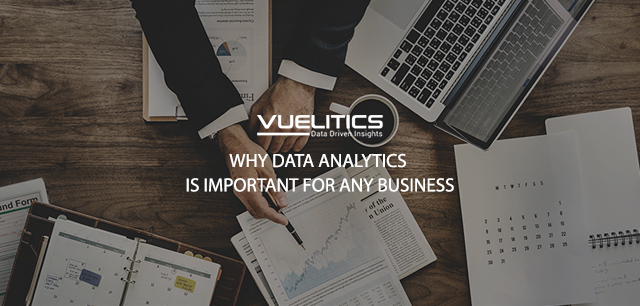
Why Data Analytics is Important for Business?
The COVID-19 situation has disrupted the business environment for the worst, and many businesses are at their wit’s end about their future. An advanced data solutions provider like Vuelitics can help executives and managers get much-needed clarity about their current businesses and prepare for the future. Most businesses are online nowadays, and the pandemic situation has forced others to do the same. Thus, data analytics in business has become extremely important, and in this article, we will discuss some of the most advanced strategies as well as the importance of data analytics.
What is Data Analytics?
Definition and Process:
Data analytics involves cleaning, interpreting, and converting raw data into actionable insights. This process helps detect patterns, relationships, and gaps, allowing businesses to make informed decisions. Whether it’s analyzing customer behavior, optimizing campaigns, or improving operational efficiency, data analytics is the key to uncovering strategic advantages.
The Importance of Data Analytics in Business
Data analytics has become a crucial part of modern business operations. It helps organizations make better decisions, optimize processes, and drive innovation. Here’s how data analytics can transform a business:
1. Informed Decision Making
Data analytics enables businesses to make well-informed decisions by providing insights into customer behavior, operational efficiency, and market trends. By identifying patterns and correlations in large datasets, companies can develop strategies that are grounded in evidence rather than intuition. This data-driven approach not only speeds up the decision-making process but also increases the likelihood of success, as decisions are based on real-world data and trends.
2. Risk management
In the volatile world of business, managing risk is crucial. Financial analytics, a subset of data analytics, plays a pivotal role in identifying potential risks before they escalate into significant issues. By analyzing historical data and current market conditions, businesses can predict potential threats and implement measures to mitigate them. This proactive approach to risk management helps protect the company from financial losses and ensures long-term stability.
3. Personalization and Customer Engagement
One of the most powerful applications of data analytics is its ability to personalize customer experiences. By analyzing customer data, businesses can tailor their interactions, recommendations, and follow-up communications to match individual preferences. This level of personalization not only enhances customer satisfaction but also fosters trust and loyalty. Customers are more likely to return to a business that understands and anticipates their needs, creating a seamless and positive experience.
4. Cost Reduction and Resource Optimization
Data analytics is a powerful tool for identifying inefficiencies and optimizing resource use. By analyzing operational data, businesses can pinpoint areas where costs can be reduced and resources can be better utilized. For instance, a study by Vuelitics revealed that data-driven decision-making in marketing can increase productivity by 15-20%, leading to significant cost savings. By optimizing processes and eliminating waste, businesses can maximize profitability and maintain a competitive edge.
5. Analyze customer behavior
In the age of the customer, understanding consumer behavior is key to staying ahead of the competition. Data analytics provides businesses with a detailed understanding of customer preferences, buying habits, and decision-making processes. Armed with this information, companies can tailor their products, services, and marketing efforts to better meet customer needs. This not only enhances customer satisfaction but also positions the business as a leader in its market, offering a superior customer experience compared to its competitors.
Data analytics is a powerful tool that helps businesses make better decisions, understand their customers, run more efficiently, and stay competitive. As data becomes more important in every industry, using data analytics effectively will be key to business success.
Additional Benefits of Data Analytics in Business
Data analytics has revolutionized how businesses operate, offering a range of benefits beyond just improving decision-making and operational efficiency. Here are some additional ways data analytics can significantly impact business success:
1. Financial Performance and Budgeting
Data analytics plays a crucial role in enhancing financial performance and streamlining budgeting processes. By analyzing financial data in real-time, businesses can gain insights into revenue trends, cost drivers, and profitability. This allows for more accurate forecasting, helping companies to allocate resources more effectively and identify areas where costs can be reduced or investments should be increased.
For instance, predictive analytics can forecast cash flow, helping businesses avoid liquidity issues by anticipating future financial needs. Additionally, by analyzing spending patterns, companies can optimize their budgets, ensuring that funds are allocated to areas that drive the most value. This level of financial oversight enables businesses to make smarter, more informed financial decisions, ultimately leading to stronger financial performance.
2. Fraud Detection and Security
In today’s digital age, fraud, and security threats are significant concerns for businesses of all sizes. Data analytics provides powerful tools to combat these risks by identifying unusual patterns or anomalies in transaction data that may indicate fraudulent activity.
Advanced analytics techniques, such as machine learning and artificial intelligence, can monitor vast amounts of data in real time, flagging suspicious activities as they occur. For example, if a sudden spike in transaction volume or unusual login behavior is detected, the system can trigger an alert for further investigation. This proactive approach to fraud detection not only helps prevent financial losses but also protects the company’s reputation.
Moreover, data analytics can enhance overall security by analyzing network traffic, user behavior, and access patterns to detect and respond to potential security breaches before they cause significant harm. By continuously monitoring and analyzing security data, businesses can stay one step ahead of cybercriminals and ensure their operations remain secure.
3. Trend Forecasting and Strategic Planning
Data analytics is a powerful tool for forecasting trends and informing strategic planning. By analyzing historical data alongside current market conditions, businesses can identify emerging trends and predict future market movements. This capability allows companies to develop strategies that are forward-looking and adaptable to changes in the market environment.
For example, retail businesses can use data analytics to predict seasonal demand for products, ensuring they have the right inventory levels at the right time. Similarly, companies can anticipate shifts in customer preferences, enabling them to adjust their product offerings or marketing strategies accordingly. This proactive approach to trend forecasting ensures that businesses are not only reacting to changes but are also prepared to capitalize on new opportunities.
In strategic planning, data analytics provides a solid foundation for setting long-term goals and developing actionable plans to achieve them. By understanding market dynamics and customer behavior, businesses can align their strategies with the trends that will drive future growth, ensuring they remain competitive in their industry.
Data analytics enables businesses to anticipate future trends and opportunities, allowing them to strategically position themselves for long-term success.
The Process of Data Analytics in Business
Data analytics in business involves several key steps:
Defining Objectives:
The first step is to clearly define the business objectives you want to achieve through data analytics. These objectives could range from improving operational efficiency to enhancing customer satisfaction or increasing revenue.
Data Collection:
Next, you need to collect relevant data from various sources. This data could be structured (e.g., databases, spreadsheets) or unstructured (e.g., social media posts, customer reviews). It’s important to ensure the quality and reliability of the data collected.
Data Cleaning and Preparation:
Raw data frequently includes errors, inconsistencies, and missing values. Data cleaning involves spotting and fixing these problems to ensure data accuracy and completeness. Preparing data involves transforming it into a suitable format for analysis, which may include normalization, aggregation, or feature engineering.
Data Analysis:
This is the core step where various analytical techniques are applied to extract insights from the data. This may involve descriptive analytics (summarizing data), diagnostic analytics (exploring relationships and patterns), predictive analytics (forecasting future trends), or prescriptive analytics (providing recommendations).
Data Visualization:
Once the analysis is performed, the data should be presented in a visually appealing and understandable format. Charts, graphs, and dashboards are examples of data visualization approaches that can assist stakeholders in better-interpreting insight.
Interpretation and Insights:
The analyzed data needs to be interpreted in the context of the business objectives. This involves extracting actionable insights and implications from the data analysis results.
Decision Making and Implementation:
Business decisions can be made to drive improvements or changes based on the insights gained from data analytics. These decisions should be aligned with the business objectives and supported by data-driven insights.
Monitoring and Iteration:
Data analytics is an iterative process. After implementing decisions, it’s important to monitor the outcomes and continuously refine the analytics process based on new data and feedback. This helps in adapting to changing business dynamics and improving decision-making over time.
Four main types of data analytics for business
Data analytics encompasses various techniques and approaches for extracting insights and value from data. Here are the main types of data analytics commonly used in business:
1. Descriptive Analytics
- Definition: Descriptive analytics focuses on summarizing historical data to describe past events and understand what has happened.
- Purpose: Provides insights into trends, patterns, and relationships in the data, helping businesses understand their current state.
- Examples: Summarizing sales data by region, visualizing website traffic over time, and generating reports on customer demographics.
Descriptive Analytics Techniques:
- Data Aggregation: Combining data from multiple sources to produce a summary.
- Data Mining: Extracting patterns from large datasets using statistical methods.
- Data Visualization: Using graphical representations (charts, graphs) to present data.
2. Diagnostic Analytics
- Definition: Diagnostic analytics aims to identify the reasons behind past events or trends by examining data correlations and causal relationships.
- Purpose: Helps businesses understand why certain events occurred and uncover the root causes of problems or successes.
- Examples: Analyzing customer churn to identify common traits among churned customers, and investigating factors contributing to a decrease in product sales.
Diagnostic Analytics Techniques:
- Drill-Down Analysis: Exploring detailed levels of data to identify patterns and causes.
- Correlation Analysis: Assessing relationships between variables to determine cause-and-effect.
- Root Cause Analysis: Identifying the primary cause of a problem.
3. Predictive Analytics
- Definition: Predictive analytics utilizes statistical algorithms and machine learning techniques to forecast future results by analyzing past business data.
- Purpose: Enables businesses to anticipate future trends, behaviors, and events, allowing them to make proactive decisions.
- Examples: Forecasting sales for the upcoming quarter, predicting customer churn, and estimating inventory levels for future demand.
Predictive Analytics Techniques:
- Regression Analysis: Modeling the relationship between a dependent variable and one or more independent variables.
- Time Series Analysis: Analyzing time-ordered data points to predict future trends.
- Machine Learning: Employing algorithms that can learn from and make predictions on data.
4. Prescriptive Analytics
- Definition: Prescriptive analytics goes beyond predicting future outcomes to provide recommendations on the actions to take to achieve desired outcomes.
- Purpose: Offers actionable insights and decision support by recommending the best course of action based on predictive models and business objectives.
- Examples: Recommending personalized marketing strategies for different customer segments, and optimizing pricing strategies based on predicted demand and competitor pricing.
Prescriptive Analytics Techniques:
- Optimization: Finding the most efficient solution to a problem by maximizing or minimizing an objective function.
- Simulation: Modeling the operation of a system to evaluate the impact of different decisions.
- Decision Trees: Using a tree-like model of decisions and their possible consequences.
Data Analytics Strategies for Business
Vuelitics primarily conducts two kinds of analysis, which are qualitative and quantitative. As the words imply, qualitative methods involve figuring out the reasons for the business’s performance. The quantitative methods involve gathering numbers and validating the reasons. However, Vuelitics also focuses on different types of approaches, such as textual analysis, statistical analysis, and diagnostic analysis. Graphical analytics using tools like Qlikview offer businesses a visual perspective, helping them easily understand complex statistics. Vuelitics specializes in predictive analysis by employing machine learning to predict patterns and trends and prescriptive analysis by suggesting numerous plans for the future.
Why choose us as your Data Analyst Partner for your Business?
Vuelitics mission is to integrate all the data points available in the client’s business using its high-performance data analytics tools and offer a comprehensive vision for business development. We specialize in guiding the business in the most profitable directions fostering optimum growth for the business. We have a systematic approach for every business that starts with a deep analysis of the business to identify the objectives and wants of the business. Equipped with the best tools in BI like Qlikview, Qliksense, and Power BI, we then chart a path to achieve those objectives and monitor the results for continuous development.
Conclusion:
Data analytics in business is going through tremendous changes and the benefits are adding up with each successive change. New trends are emerging that can effectively cut losses and accelerate profits for any business. Thus businesses with a data solution partner like Vuelitics have a competitive edge over their peers.
Ready to unlock the full potential of your business with data analytics? Partner with Vuelitics, your trusted Business Intelligence and Analytics service consultant, and transform your raw data into actionable insights that drive growth, efficiency, and profitability. Contact us now to discover how our advanced data solutions can give you the competitive edge you need in today’s dynamic market. Let Vuelitics guide you toward a data-driven future!
Recent Posts
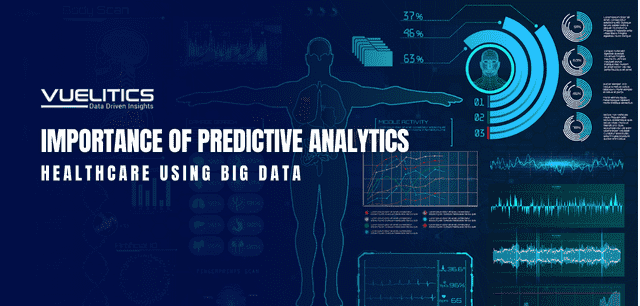
Importance of Predictive Analytics in Healthcare Using Big Data
2 September, 2024
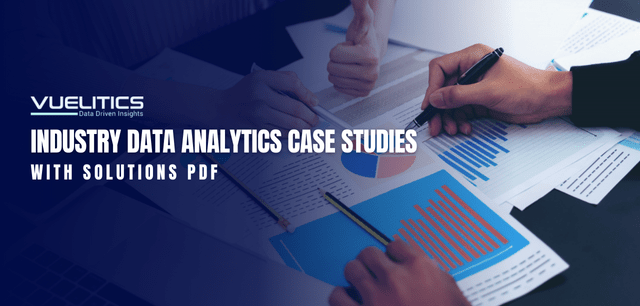
Industry Data Analytics Case Studies with Solutions PDF
29 August, 2024
Post Comments
BI & Data Analytics: Transform Your Business Strategy Now.
July 25,2024
[…] Read More: Data Analytics in Business: Why is It Important? […]
Business Intelligence Technologies: Stay Ahead of the Competition
July 02,2024
[…] Artificial intelligence (AI) uses computer systems to simulate different attributes of human intelligence, like learning, problem-solving, and decision-making. The sophistication with which it can model human intelligence and extract insightful data from reports makes it an important thing in business data analytics. […]